KBY-AI’s face liveness detection, face anti-spoofing algorithms are applying SOTA artificial intelligence-based methods, including machine learning and deep Learning algorithms which have been discussed in this blog below for mitigating biometric fraud.
Biometrics has been evolving as an exciting yet challenging area in the last decade. Though face recognition is one of the most promising biometrics techniques, it is vulnerable to spoofing threats. Many researchers focus on face liveness detection to protect biometric authentication systems from spoofing attacks with printed photos, video replays, etc.
As a result, it is critical to investigate the current research concerning face liveness detection, to address whether recent advancements can give solutions to mitigate the rising challenges.
This face liveness detection research performed a systematic review using the PRISMA approach by exploring the most relevant electronic databases.
The article selection process follows preset inclusion and exclusion criteria. The conceptual analysis examines the data retrieved from the selected papers.
To the author, this is one of the foremost systematic literature reviews dedicated to face liveness detection that evaluates existing academic material published in the last decade. The research discusses face spoofing attacks, various feature extraction strategies, and Artificial Intelligence approaches in face liveness detection.
Introduction Of Face Liveness Detection Approaches
Biometric authentication has consistently outperformed conventional password based authentication schemes. Personal identification was limited in prehistoric
times.
Today, computer vision and biometrics can distinguish people without credentials or artifacts. Biometrics can identify people instead of their affiliations, belongings, or confidential information. The need for accurate and machine-based identification led us to biometrics, which uses technology to speed up the process of identifying and authenticating people. The printed IDs have been replaced with biometric IDs, which allow for proof of ‘who you are’ without carrying a card or other document.
Biometrics have been intensively researched for their automation, accessibility, and precision in meeting the increasing security demands of our daily life. As the technology has evolved through monitoring crime identification and forensics, it is a machine that analyzes human individuals’ physiological and behavioral characteristics to classify them uniquely.
As per a report by (accessed on 16 January 2023
), the market of contactless biometrics would reach 37.1 billion USD whereas, by 2028, the face-based biometric recognition market would reach USD 12.11 billion due to promising applications in diverse categories, as given in the “Facial Recognition Business” report.
Biometrics has been effectively implemented in several areas where security is a top priority. For instance, personal identity cards for airport checkin and check-out, confidential data from unauthorized individuals, and credit card validation.
Several biometric features, including fingerprint, iris, palm print, and face, are utilized for recognition and authentication. Face-based authentication provides more secure contactless authentication of the user than fingerprints and iris.
However, one of the biometric recognition systems’ most significant challenges is deceptive identification, widely known as a spoofing attack. Submitting a facial artifact of a legitimate user could easily construct using a person’s face photos or videos from a “public” social media platform; an impostor can quickly access an insecure face recognition system.
In general, also referred to as presentation attacks, these are straightforward, easy to implement, and capable of fooling face recognition (FR) systems and providing access to unauthorized users. These are becoming critical threats in advanced biometric authentication systems. Effective face liveness detection systems are increasingly attracting more attention in the research community, and several challenges make it difficult.
Significance and Relevance
A few biometric traits evolved as the field progressed and occasionally disappeared. To be sure, face recognition is one biometric characteristic that has stood the test of time. Face characteristics are distinctive. Face-based authentication offers a more reliable yet contactless user identity than iris and fingerprint scanning.
Face biometrics, which provides a secure identity and forms the basis of an inventive biometric system, has thus emerged as the preferred study area. However, printed face images or other artifacts can be used to fake invader challenges on face biometric systems, making them highly vulnerable. Spoofed faces can stop the face recognition system from working correctly.
Various researchers concentrate on identifying facial liveness to prevent attacks on the biometric system.
Therefore, it is crucial to categorize the current research on the biometric of face liveness detection to address how growing technologies might provide explanations to lower the emerging hazards. Facial recognition-based applications have made tremendous progress due to artificial intelligence (AI) techniques. Deep learning has advanced in recent years.
The use of artificial neural networks or convolutional neural networks (CNNs) in many computer vision tasks has been extensively studied, especially with the advancement of robust hardware and enormous datasets.
Image categorization and object detection were successfully solved using CNNs. The existing body of literature on face liveness detection concentrates on advances in hardware and software and various categorization methods employing ML- and DL-based methodologies. It is essential to do a comparative examination of these procedures based on several assessment criteria.
It is necessary to thoroughly examine the pertinent articles and academic publications to understand what research has been directed toward biometric and face liveness detection. This study seeks to provide information on a range of datasets, performance metrics, face spoofing attacks, and methods for detecting the liveness of a face.
Image Quality Assessment Advanced algorithms evaluate factors like lighting, focus, and facial positioning to ensure the captured image meets the necessary standards for its intended use, such as identity verification or liveness detection.
Image quality is an important aspect in face recognition. But being too demanding on image quality during the face capture can also impact user experience. Therefore, the application should only require sufficient quality inputs for the specific use case in question.
For example, login should be quick and require only basic adjustments, as opposed to passport quality image capture where correct lighting and background uniformity are required. Quality can be controlled by various quality attributes, but to simplify integration, pre-configured quality providers are available to cover most common use cases.
Evolution of Face Biometric Liveness Authentication
Fingerprints and other biometric features were used in previous biometric identification research. Semi-automated facial recognition systems that were distinctive to
each person were initially proposed in 1988. Early in 2010, a face liveness detection algorithm was created. Since 2013, Face Liveness Detection (FLD) research has extensively used machine learning (ML) technologies.
The potential of ML to forecast and classify data is a key justification for using these algorithms. The face liveness detection techniques include logistic regression, SVM, AdBoost, and Random Forest. The progress of face biometric authentication is seen in Figure below.
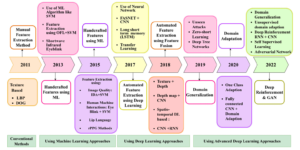
Huge volumes of information are processed using deep learning (DL) algorithms. The researchers started utilizing deep learning technology when facial liveness detection algorithms were introduced. Researchers have adopted DL methods for face liveness identification because they offer superior features to conventional handmade features.
Some academics began working on the pre-trained networks used for face liveness detection, including convolutional neural networks (CNN), ResNet50, Inception model, VGG16, VGG19, GoogleNet, and AlexNet.
Research Methodology
A systematic review was carried out using the Preferred Reporting Items for Systematic Reviews and Meta-Analysis (PRISMA) process. A set of guidelines for conducting systematic reviews and other data-driven meta-analyses are given in PRISMA Approach.
The conduct of a systematic review three steps protocol is used in this paper: the formulation of research questions, the search databases, and the criteria for inclusion and exclusion of research articles—the details of these steps for research analysis are detailed in the following sections.
This systematic review is organized to cover the study’s breadth under consideration by categorizing and evaluating existing publications. The first step is to define the research questions so that the coverage rate of current works is accurately described. There should be some perspectives that can help researchers generate new ideas by analyzing similar results.
The next step is to develop procedures for reviewing the technical and scientific articles that these searches produced to identify relevant papers.
The proposed approach is divided into two phases. The first phase uses Boolean operators AND/OR to identify search terms from research questions and prepare a list of keywords. The second phase uses Boolean operators AND/OR to select queries to search for and collect all relevant data. Table below gives the list of fundamental, Primary & Secondary Keywords.
Conduction of SR
The authors utilized the following steps to choose appropriate papers for this research: As represented in the PRISMA flow diagram, the measurements of identification, screening, eligibility, and inclusion. Figure 3 depicts a PRISMA (Preferred Reporting Items for Systematic Review and Meta-Analysis) flowchart diagram showing the
identification and records selection process of studies for the systematic review. In figure below, # is used to indicate the process of screening is followed. Scopus and Web of Science (WoS) are well-known and standard research databases for searching the query.
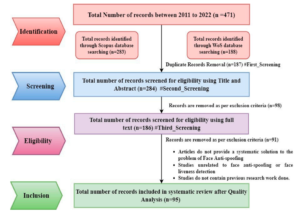
Two hundred eighty-three research articles from Scopus and 188 from WoS are retrieved using a search query. A total of 471 records are further gone through the first screening process of duplicate records removal. One hundred eighty-seven duplicate records are removed based on doi and title.
Later, 284 documents were undergone through a second screening process using inclusion and exclusion criteria of the title and abstract.
Further, 98 documents still need to meet the inclusion criteria. Hence, 186 papers are forwarded to check for eligibility criteria. Based on inclusion and exclusion criteria for full text, the number of documents included for review is 95. Authors critically review these 95 research articles to find the research gaps in the existing literature and future directions in facing anti-spoofing.
Face Spoofing Attacks
With the advancement in technology, facial recognition systems have increased so widely. Along with that, the challenges or threats to facing recognition systems also come into the picture. Intruders use various spoofing techniques to fool the facial recognition-based authentication systems, known as Face Spoofing attacks and are also commonly termed Face presentation attacks.
The different types of spoofing techniques or attacks are discussed in this section. Face Spoofing attacks are classified as shown in Figure below.
The face spoofing attacks are broadly categorized as static attacks & dynamic at tacks, whereas those are sub-classified by 2D or 3D static & dynamic attacks.
2D Static & Dynamic Attacks
2D Static Spoofing attacks are when intruders employ pictures, flattened papers, and masks for authentication. Images taken on paper are kept in front of the face recognition system by intruders to get access to the systems. A paper should be of good quality and in the A3 or A4 size. Printed picture attacks are one type of printed photo attack. It is the most common type of attack as it is easy to perform due to the large availability of individual pictures on social media.
Another approach to spoof images is to cut a printed photo on the eyes or lips region to add some liveliness to the photo kept in front of the camera; this is known as a cut photo at tack, as shown in Figure below.
As face recognition-based systems get prone to attacks, in truders also come with different attacks. Another method for creating spoof photo graphs is to hold a genuine user’s photo in front of the camera in a tilted position, either horizontally or vertically, to give the image depth.
The matte paper and printed masks, plastic masks, are used as attacking instruments. 2D static attacks are quick and straightforward to execute in actual circumstances, necessitating sophisticated facial recognition algorithms.
Furthermore, a high-definition photo on a smartphone screen, a series of high definition images, and a video of a genuine person on the screen of smart and portable devices shown in front of the camera of face authentication systems are other approaches to spoofing attacks. These attacks are 2D dynamic attacks.
Video replay at tacks are superior to 2D static photo attacks because they incorporate unique features such as eye movements, lip motions, and shifts of facial emotions to simulate liveness.
Replay attacks are often harder to spot than photograph spoofs since they imitate the texture and contour of the face and its dynamics, such as eye movements, lips, and facial expressions.
Facial recognition systems vulnerable to 2D static picture attacks would perform significantly worse against 2D dynamic video attacks.
Being robust against photo at tacks can mean something other than being similarly strong against video attacks. As a result, appropriate measures should be designed and implemented for robust face recognition systems.
3D Static & Dynamic Attacks
3D static & dynamic attacks provides a 3D mask of the person’s face. The attacker creates a three-dimensional reconstruction of the victim’s face and shows it to the camera/sensor. Mask attacks involve more significant expertise than 2D static and dynamic attacks and access to additional facts to generate an accurate mask of a legitimate user.
3D static attacks employ a 3D mask as an instrument of 2D images adhered to a flexible structure such as a shirt or plastic bag. Such attacks fool low-level 3D face recognition systems.
Different Sculptures are also used as attacking instruments in 3D static at tacks. Two or more photos of the actual user’s face, such as one frontal shot and one profile shot, can be used to create 3D models. The attacker may be able to extrapolate a 3D reconstruction of the real face using these images.
Another type of 3D attack is a makeup presentation (M- PA) attack. For impersonation, the attacker may use heavy makeup to mimic the facial look of a target subject.
3D masks present additional hurdles to the FR system; the Multi-Modal Dynamics Fusion Network (MM-DFN) technique is being investigated. It gets more challenging to create effective counter measures as the entire face structure is copied. 3D mask attacks are predicted to become increasingly common in future years as 3D acquisition sensors become more ubiquitous.
Artificial Intelligence for Face Liveness Detection
The various face liveness detection AI-based techniques discussed in the literature are categorized based on the ML approach & Deep Learning Approach. Studies reveal that artificial intelligence-based methods for detecting face presentation attacks are frequent.
This systematic review examined various academic research articles on face presentation attack detection using AI-based techniques for face liveness detection.
The authors answered research questions through a systematic review using the PRISMA protocol. Such as varied challenges and problems associated with the different face spoofing attacks, datasets, face presentation at- tack detection using artificial intelligence-based approaches used for robust face recognition systems, and future directions as follows.
Various feature extraction methods, such as handcrafted feature-based extraction, texture-based, motion-based, and depth-based methods, and the fusion of multiple feature extraction methods, are proposed in the literature.
Face liveness detection systems use a combination of machine learning and deep learning algorithms to achieve optimum accuracy in seeing false and real faces. Even while these approaches offer correct findings for the existing types of attacks, they are vulnerable to invisible attacks or attacks that are unable to detect by the systems.
Due to their lack of generalization capacity, existing approaches cannot detect invisible attacks (such as synthetic faces). Public datasets are still a long way from accurately reproducing real-world applications. It is likely owing to the difficulties of gathering PAs and PAIs from impostors in the wild. As a result, most PA examples are obtained manually, which is time consuming and exhausting.
Moreover, generating a large-scale dataset for face anti-spoofing in the wild covers numerous real-world applicative contexts. When faced with these challenges, some researchers rely on data augmentation techniques to create synthetic (yet realistic) photographs of PA.
Face presentation attack detection (FAD) problems use various artificial intelligence-based approaches. Due to AI-based advancements, the classification of fake or real spoofs for robust face recognition systems has become possible. Literature looks at AI-based models, such as convolutional neural networks, transfer learning, domain adaptation, domain generalization, generative adversarial network, reinforcement learning, explainable AI, etc.
The handcrafted feature-based face liveness detection methods are considered to be more robust against the unknown data samples (data samples not being considered during training of the AI-based face FLD techniques).
The deep learning stacks of neural networks are more reliable in performance, especially in cases where training and testing data samples are very similar. For unknown attacks such as synthetic faces (artificially created), the fusion of handcrafted and auto-extracted (through deep learning) features may perform promisingly with the help of AI techniques.
In the future, to improve the robustness of existing face liveness detection techniques, domain adaption, and generalization strategies, investigate further to deal with undetected or unseen attacks.
To make existing algorithms more robust, considering employing multiple algorithms together for decision-making using either federated learning or decision fusion would be the further research direction in the field.
Frequently Asked Questions
What is face liveness detection?
Face liveness detection is a technique used to determine if a captured face is from a real, live person or an artificial representation like a photo, video, or mask. This ensures the authenticity of facial data during biometric verification.
How does AI improve face liveness detection?
AI leverages machine learning and deep learning models to analyze subtle details such as micro-expressions, texture patterns, and depth information. This enables more accurate detection of live faces and prevents spoofing attacks.
What are the common methods used for liveness detection?
Common methods include static image analysis, video-based analysis, depth sensing and AI-driven models.
Can face liveness detection systems work offline?
Yes, some face liveness detection systems can work offline if they are designed with pre-trained AI models. However, cloud-based solutions are more common for handling large-scale and real-time data processing.
Where can on-premise face liveness detection be found?
Just reach out to KBY-AI company, which is powerful identity verification SDK provider.
Conclusion
Face liveness detection using biometric identification techniques is widely used nowadays but has many potential threats.
The research also finds a high-quality publicly available real-world dataset for 2D and 3D spoofing attacks, which would be the foundation for developing robust FPAD systems. It discussed challenges as well as future research goals in the domain of invisible attack detection methods.
However, several unique challenges must be addressed before they are employed in real-world face recognition-based authentication systems. Although most current solutions rely on handcrafted features to combat presentation attacks, machine learning and deep learning-based feature extraction methods are also used.
Although existing approaches perform better intra-testing, they lack generalization capabilities, which is crucial for unknown threats. In the real-world implementation of face liveness detection systems, domain adaptation, and explainable artificial intelligence need to be explored.
These discoveries could serve as a foundation for future research toward face liveness detection that is secure and robust. The findings of this study would undoubtedly guide the biometric sector in developing safe, efficient, and trustworthy face liveness detection systems in the coming years of biometric technological development.