Face anti-spoofing techniques from KBY-AI include texture analysis and liveness detection methods. These approaches ensure biometric facial recognition systems are tamper-proof.
In the realm of digital security, safeguarding biometric systems against spoofing attacks is paramount. Face anti-spoofing technologies have therefore become essential for authenticating individuals without being fooled by masks, photos, or video impersonations. These methods leverage advanced algorithms and artificial intelligence to analyze the uniqueness of a human face in real-time.
By examining the skin texture, analyzing facial expressions, and detecting involuntary human traits such as eye blinking or subtle facial movements, these systems can effectively differentiate between a real person and a fraudulent attempt to gain access. Implementing these robust security measures is critical in a variety of sectors including banking, secure facility access, and mobile device unlocking, reinforcing trust in biometric verification.
Introduction To Face Anti-spoofing
The Rise Of Biometric Security
The adoption of biometric security measures is skyrocketing. Unique human traits like fingerprints, iris patterns, and facial features are now keys to our digital world. As the reliance on these personal attributes intensifies, the need for stringent security measures becomes undeniable.Challenges In Ensuring Facial Recognition Integrity
Ensuring the integrity of facial recognition technology is fraught with challenges. Advanced face anti-spoofing strategies from KBY-AI aim to overcome these hurdles. These strategies involve sophisticated techniques to safeguard against deceptive practices such as photo, video, or mask-based frauds. The table below summarizes common threats and the corresponding face anti-spoofing solutions.Type of Spoof | Anti-Spoofing Solution |
---|---|
Photo Attacks | Liveness Detection |
Video Replay Attacks | Motion Analysis |
3D Mask Attacks | Texture and Depth Analysis |
Types Of Face Spoofing Attacks
Photo And Video-based Attacks
These are simple, yet effective ways to fool a system. Attackers use a photo or video of a valid user to gain entry. This method exploits systems that are not advanced enough to spot the difference between a real person and an image.- Print Attacks – Using a printed photo of the user.
- Replay Attacks – Playing a video of the user.
- Mobile Displays – Showing a photo or video on a mobile screen.
3d Masks And Dolls
Criminals sometimes use 3D masks and dolls. These look real and can trick even advanced systems. These attacks use masks or sculptures that replicate a person’s facial features in three dimensions.Attack Method | Description |
---|---|
Silicone Masks | Masks created with lifelike materials. |
Realistic Dolls | Dolls modeled to resemble a person. |
Deepfakes And Digital Manipulation
Deepfakes use AI to create false images and videos. They can be very convincing. This method involves digitally altering video footage and images to create the illusion of a real person’s likeness, often indistinguishable from reality.- Creating a digital face that mimics the user.
- Altering existing video to add or change facial movements.
KBY-AI’s Approach To Anti-spoofing
KBY-AI‘s Background And Expertise
KBY-AI boasts a team of world-renowned experts in artificial intelligence. They specialize in biometric security. Their achievements include groundbreaking work in machine learning, computer vision, and cybernetic security. This expertise has driven them to develop high-grade anti-spoofing measures, safeguarding against unauthorized access through facial recognition systems.Innovative Solutions For Face Verification
The company’s suite of anti-spoofing tools are both robust and user-friendly. They encompass a range of techniques, from liveness detection to 3D facial mapping. Below is a breakdown of their innovative approaches:- Real-time liveness detection: Ensures the presence of a live person instead of a photo or video.
- Texture analysis: Detects anomalies in skin patterns that could indicate a mask or synthetic skin.
- 3D depth analysis: Uses structured light or time-of-flight sensors to capture the contours of a face, ensuring its physical presence.
- AI-driven behavior analysis: Assesses micro-movements and expressions for signs of natural human behavior.
- Multi-spectral image analysis: Applies invisible light to uncover hidden details not seen in standard photos or videos.
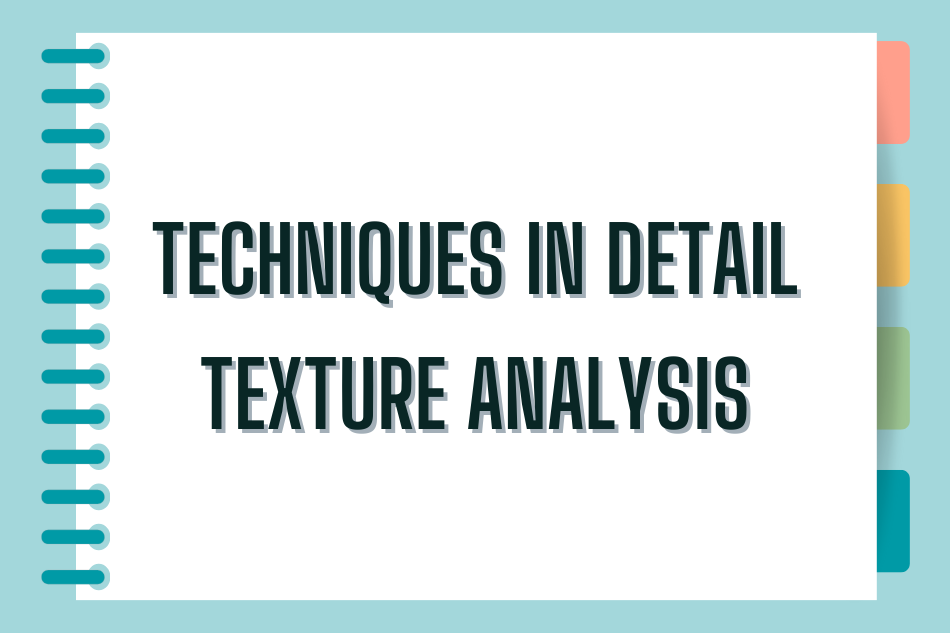
Techniques In Detail: Texture Analysis
Examining Micro-textures
The human face has unique micro-textures which fraudsters find hard to replicate. KBY-AI deploys high-resolution analysis to detect these fine patterns. The process involves:- Zooming in on facial features to reveal micro-texture details.
- Utilizing algorithms to compare these details against known genuine textures.
- Highlighting discrepancies that indicate potential spoofing attempts.
Detecting Surface Reflectivity
Surface reflectivity differs between real skin and synthetic materials. KBY-AI harnesses this fact for authentication. Their method comprises:- Analyzing how light reflects off the face.
- Assessing the reflectance for natural inconsistencies.
- Flagging abnormal reflection patterns that may suggest a fake face.
Criteria | Genuine Texture | Spoof Texture |
---|---|---|
Detail Level | High-resolution, complex patterns | Simple, often blurred imitations |
Reflectivity | Unpredictable, natural variances | Uniform, may lack luster |
Detection Method | Advanced algorithms, deep learning | Comparative analysis, consistency check |
Frequency-based Methods
Exploring FFT And LBP Techniques
Two standout techniques in the frequency-based arsenal are Fast Fourier Transform (FFT) and Local Binary Patterns (LBP). FFT transforms a facial image into the frequency domain to reveal patterns invisible in the time domain. LBP, on the other hand, encodes the texture of an image by comparing each pixel with its neighborhood. It creates a robust descriptor of the face that’s less sensitive to variations in lighting or pose. Implementing FFT: FFT breaks down images into sine and cosine components. Our systems swiftly calculate these elements to expose inconsistencies typical in spoof attacks. Utilizing LBP: By analyzing the unique texture patterns on genuine faces, LBP can effectively flag abnormal texture distinctions indicative of a spoof attempt.- FFT pinpoints frequency anomalies
- LBP targets texture-based irregularities
Resilience Against Highly-realistic Masks
The rise of lifelike masks poses a genuine threat to security. Frequency-based methods like FFT and LBP are particularly adept at identifying the subtleties that differentiate a real human face from a high-fidelity mask.Technique | Advantage |
---|---|
FFT | Detects abnormal frequency patterns often found in masks |
LBP | Highlights inconsistencies in surface texture not present in real skin |
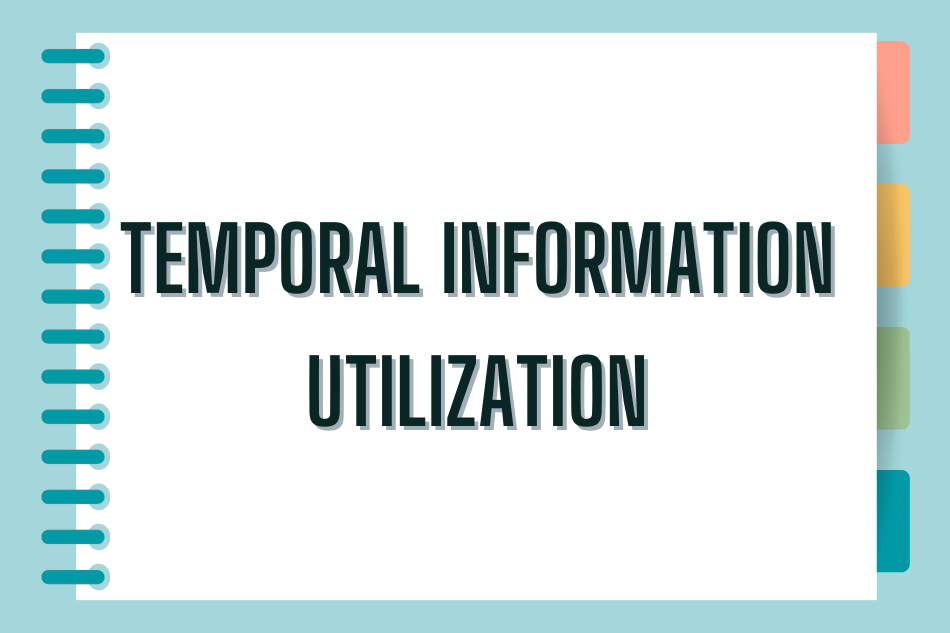
Temporal Information Utilization
Analyzing Motion And Behaviour
Temporal data shines when analyzing motion and behavior. KBY-AI deploys advanced algorithms that track facial expressions and head movements. This approach captures how a real person’s face naturally changes over time. Real-time analysis identifies authentic human interactions, leaving imposters behind.- Head rotation: Tracks the angle of head movements.
- Blinking patterns: Observes natural blinking frequency.
- Expression transitions: Notes how expressions evolve.
Capturing Subtle Facial Dynamics
Beyond obvious movements, KBY-AI digs deeper. The technology focuses on subtle facial dynamics not easily replicated by fakes. These nuances are a telltale sign of living, breathing humans. The system meticulously records minor shifts in expression and uses this data to bolster security measures against spoofing.Facial Feature | Details Captured |
---|---|
Eyes | Micro-movements and twitches |
Mouth | Subtle lip movements and twitches |
Deep Learning Strategies
Convolutional Neural Networks (CNN’s)
Convolutional Neural Networks excel in image recognition and processing. They mimic the human visual cortex to identify complex features in facial images. CNNs can distinguish between a real face and a mask or a photo. Kby-Ai leverages multi-layered CNNs to analyze texture patterns and other facial indicators that signal a spoof attempt. This process involves several key steps:- Image Preprocessing: Prepares images for analysis.
- Feature Extraction: CNNs learn to identify important facial features.
- Classification Layers: They predict whether an input is genuine or fake.
Recurrent Neural Networks For Sequential Data
Recurrent Neural Networks (RNNs) are ideal for data that comes in sequences. Unlike static images, some spoofing attacks use videos or motion. This is where RNNs shine in the context of face anti-spoofing. They process video frames over time, detecting inconsistencies and irregularities in movement that could indicate a spoof. Key features of RNNs include:- Temporal Analysis: RNNs consider the timing between video frames.
- Memory Elements: They remember past information, crucial for spotting fakes.
- Sequential Processing: Each frame is analyzed in context, not in isolation.
Liveness Detection Protocols
Active User Interaction
Active user interaction asks people to do something to prove they are real. Like moving their eyes or smiling. This check makes sure the person in front of the camera is live and not a photo or video.- Show your face
- Turn your head
- Blink your eyes
Passive Detection Using Environmental Cues
Now, let’s get smart without any work from the user. Passive detection uses clues from around you. It can tell if the room is light or dark, or if the face in front of the camera moves with normal life-like motion. No need for smiles or blinking here. The camera and software do the work, checking things like:What it sees | What it means |
---|---|
Light from the room | Is it natural or artificial? |
Shadow changes | Does the shadow move like a real person’s would? |
Face depth | Proves the face isn’t flat like a photo. |
Hybrid Models
Combination Of Multiple Techniques
Hybrid models don’t rely on a single method. This approach mixes various anti-spoofing techniques to cover a wide range of attack scenarios.- Texture Analysis: Detects patterns that are not present in genuine faces.
- Frequency Response: Uses signal processing to find unnatural frequency components.
- 3D Face Shape: Assesses the depth information to confirm the face is not a flat image.
- Behavioral Analysis: Recognizes natural human motion and response.
Enhancing Accuracy And Robustness
Accuracy and robustness are crucial in anti-spoofing technology. Hybrid models enhance these attributes significantly.Feature | Benefit |
---|---|
Multimodal Data | Increases the potential data points for authentication, improving security. |
Machine Learning | Refines detection capabilities over time, making the system smarter. |
Real-time Processing | Ensures immediate response to spoofing threats, safeguarding users. |
End-to-end Systems
Integration With Biometric Authentication
Face anti-spoofing protects against fake access attempts. KBY-AI’s technology seamlessly integrates with various biometric systems. It adds an extra security layer without compromising the established authentication flow.- Enhanced Security: By working alongside fingerprint and iris scans, face anti-spoofing makes security tighter.
- Adaptive Algorithms: KBY-AI’s models adapt to different biometric features, creating a robust system.
- Real-Time Verification: Instant checks provide immediate feedback on authentication attempts.
User Experience Considerations
User comfort is key. KBY-AI’s anti-spoofing technology ensures security without sacrificing convenience. Smooth interactions and intuitive design keep users satisfied.Aspect | Importance |
---|---|
Speed | Users expect quick verification processes. |
Accuracy | High precision in detecting spoofs is critical. |
Simplicity | Easy-to-use interfaces encourage consistent use. |
Testing And Evaluation Metrics
Benchmark Datasets
For rigorous testing, various benchmark datasets play a pivotal role. These datasets provide standard challenges consisting of various spoofing attacks. Developers and researchers use them to test and improve anti-spoofing algorithms.- Live Face Detection Dataset (LFDD): A collection of real-world scenarios.
- Replay-Attack Dataset: Contains videos with replay attacks to test temporal-based systems.
- 3D Mask Attack Dataset (3DMAD): Provides 3D mask spoof attack scenarios.
Performance Indicators And Comparison
Key performance indicators enable comparison across different anti-spoofing solutions. Researchers look at accuracy, speed, and error rates.Indicator | Description |
---|---|
True Positive Rate (TPR) | Measures the correct identification of genuine users. |
False Positive Rate (FPR) | Indicates the rate of false alarms in recognizing spoof attempts. |
Equal Error Rate (EER) | Point where false acceptance rate and false rejection rate are equal. |
Accuracy | Overall effectiveness of the system in all conditions. |
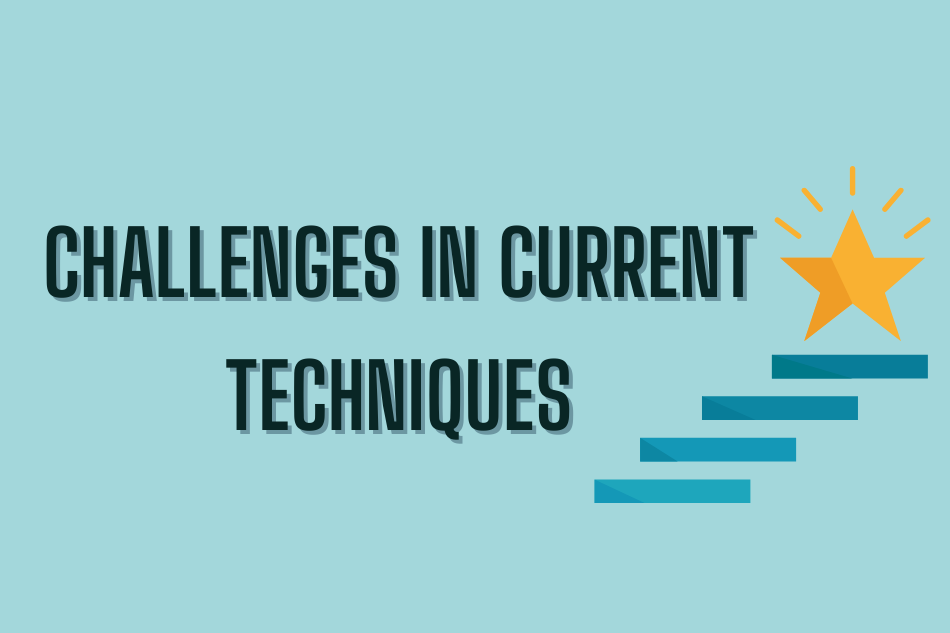
Challenges In Current Face Anti-Spoofing Techniques
Addressing Varying Light And Environmental Conditions
One major challenge is changing light and settings. Good anti-spoofing must work anytime, anywhere. This is tough. Lights change. So do backgrounds. Anti-spoofing tech must adapt.- Indoor vs outdoor – Tech must handle both.
- Changing shadows and brightness affect detection.
- Cameras differ – Anti-spoofing must be universal.
Dealing With Diverse Attack Vectors
Attackers use many tricks. They might use photos, videos, or masks. Tech must catch them all. This means always learning new patterns.Attack Vector | Challenge |
---|---|
Photos | Simple to use but should be easy to spot. |
Videos | More complex because they mimic real movement. |
Masks | Highly realistic masks are tougher to detect. |
Real-world Applications And Case Studies
Finance And Banking
In the finance and banking sector, safety means everything. With face anti-spoofing technology, banks safeguard clients’ accounts. Let’s explore some practical uses.- Mobile Banking: Face anti-spoofing ensures the person accessing the app is the actual account holder.
- ATM Transactions: Modern ATMs use facial recognition to authenticate users, reducing card fraud.
- Online Applications: Equipped with these techniques, banks verify customers’ identity remotely with high accuracy.
Border Control And Airport Security
At borders and airports, security cannot tolerate compromises. Face anti-spoofing provides an extra layer of protection.- E-Passport Gates: These use advanced face verification to speed up the process, while ensuring security.
- Surveillance Systems: High-risk areas employ these techniques to flag fraudulent activities in real-time.
- Boarding Procedures: Airlines implement face recognition, cutting down wait times and preventing identity fraud.
Legislation And Privacy Concerns
Regulatory Frameworks
Global strategies ensure face anti-spoofing tools abide by laws. These frameworks outline the use and limits of biometric data. They include:- GDPR in Europe, safeguarding personal data.
- The Biometric Privacy Act in the USA, controlling biometric use.
Balancing Security And User Privacy
The goal is simple: maximize security while protecting privacy. Achieving this involves:- Leveraging technology that respects user consent.
- Transparency about data collection.
- Robust data protection measures.
Adaptive Algorithms For Continuous Learning
Machine Learning That Evolves With Threats
Machine learning is the brain of anti-spoofing. KBY-AI’s technology is like a guard that learns. Every new trick teaches it to be stronger. The system changes as threats do. It never stops getting better. Think of it as a smart watchguard that grows with every attack it faces.- Real-time analysis for quick adjustments
- Automated updates keeping defenses sharp
- Pattern recognition that outsmarts fakes
Incorporating New Data Into Models
Kby-Ai makes sure new information doesn’t go to waste. The team feeds new data into their algorithms regularly. This is key for staying ahead. Their models absorb fresh data like sponges.- Collect new examples of spoofing attempts
- Analyze and learn from these attempts
- Improve the detection capabilities
The Human Factor In Anti-spoofing
Face anti-spoofing is crucial in this digital age. It keeps our devices and data safe. But, technology alone can’t catch everything. People are a key part of keeping security strong.
Training Staff On Security Protocols
Every team member must know about security threats. Training helps them spot and stop attacks. Here’s a simple plan to do that:
- Regular workshops: Keep your team updated on the latest threats.
- Clear guidelines: Give simple steps on what to do during a threat.
- Hands-on practice: Let staff try anti-spoofing tools themselves.
- Quiz them: Check their knowledge with quick tests.
Human Oversight In Automated Systems
Robots are smart, but they need us. Here’s how we can keep an eye on things:
- Check alerts: Staff should review security alerts.
- Understand patterns: Know what normal activity looks like. Spot the odd ones out.
- Decision power: People should make the big calls on suspicious activities.
Remember, the right mix of people and technology makes the best shield against fakes.
Future Of Face Anti-spoofing Technology
Predicted Technological Advancements
Experts believe the future holds incredible innovations for face anti-spoofing. Let’s explore some of these advancements:- 3D Face Mapping: This will create deep layers of facial recognition, making deceiving systems more challenging.
- AI and Machine Learning: Algorithms will self-improve, predicting and preventing new spoofing attacks.
- Multi-Sensor Authentication: Combining multiple sensors strengthens security and reduces false acceptance rates.
Potential Shifts In Biometric Security
Biometric security is not static. The field is set for several shifts, including:Shift | Impact |
---|---|
Behavioral Biometrics: | User behavior patterns add an extra layer of security. |
Adaptive AI Systems: | Systems will learn from attempts at deception to become smarter. |
Privacy-Preserving Techniques: | New methods will protect user data during the authentication process. |
Comparative Analysis Of Industry Solutions
KBY-AI Vs. Other Anti-spoofing Technologies
KBY-AI is a standout in the realm of face anti-spoofing with its cutting-edge solutions. Let’s compare it to others.Feature | KBY-AI | Other Technologies |
---|---|---|
Machine Learning | Advanced deep learning | Varies across solutions |
Speed | Real-time checks | Often slower |
Accuracy | High precision rate | Inconsistent rates |
User Experience | Seamless integration | Can be intrusive |
Market Leaders And Emerging Players
The anti-spoofing market parades a mix of established leaders and emerging players. Below is a list identifying them:- Market Leaders:
- FaceID by Apple
- Google Tensorflow
- SenseTime
- Emerging Players:
- KBY-AI
- DeepFake Detection Challenge players
- Startup ecosystems’ newcomers
Adapting To New Normals
Post-pandemic Security Requirements
The pandemic has reshaped how we think about security and personal interaction. Organizational structures now require robust security measures that can be deployed remotely and ensure the health and safety of individuals.- Face recognition: a vital tool for safe, remote identification.
- Biometric solutions: adapted to limit physical touchpoints.
- KBY-AI’s advanced algorithms: cater to these evolving needs.
Ensuring Contactless Yet Secure Authentication
In a world where contactless interactions are preferred, secure authentication is paramount. KBY-AI’s technology steps up to this challenge.Feature | Benefit |
---|---|
Real-time processing | Quick verification with no delays |
Liveness detection | Prevents spoofing attacks |
Zero physical contact | Supports current health guidelines |
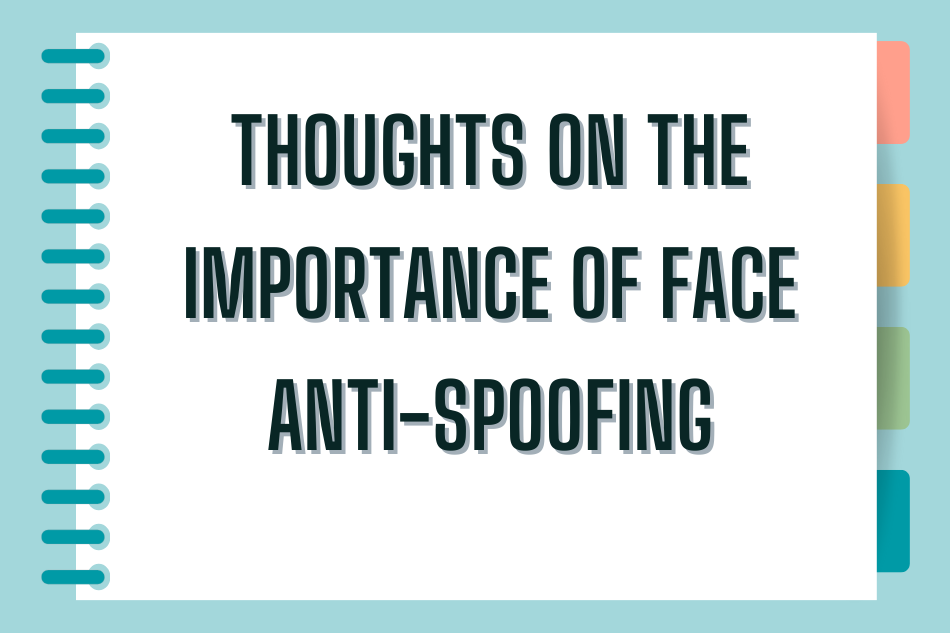
Concluding Thoughts On The Importance Of Face Anti-spoofing
Summarizing The Need For Robust Anti-spoofing
Identity theft is no joke. Cybersecurity threats are growing. We need better face anti-spoofing to protect us. These tools check if a real person or a photo is trying to gain access. They use special algorithms and sensors to tell the difference.- Spoofing attacks can be photos, videos, or masks.
- Anti-spoofing tools analyze depth, texture, and response to light.
- They keep your devices and accounts safe.
Future Directions And Final Remarks
Face anti-spoofing will get smarter. Researchers at KBY-AI are working hard on this. They are finding new ways to spot fakes better and faster.Current Capabilities | Future Improvements |
---|---|
Texture analysis | Improved 3D modeling |
Liveness detection | Live skin analysis |
Depth sensing | Subtle motion detection |
Note for implementation: Ensure the actual HTML implementation uses correct tagging instead of the escaped < and > for the H3 tags provided just as a visual representation.
Frequently Asked Questions Of Face Anti Spoofing Techniques From KBY-AI
What Is Face Anti-spoofing?
Face anti-spoofing is a security measure used to prevent unauthorized access by distinguishing between a live person’s face and a fake representation.
How Does KBY-AI Enhance Security?
KBY-AI enhances security by implementing advanced algorithms to detect and thwart facial recognition spoofing attempts.
Can KBY-AI Prevent Photo Attacks?
Yes, KBY-AI’s technology is designed to identify and block attempts to use photographs to bypass face authentication systems.
What Makes KBY-AI Reliable For Anti-spoofing?
KBY-AI’s reliability stems from its use of cutting-edge machine learning techniques and continuous database updates to stay ahead of spoofing tactics.
Does KBY-AI Support Live Detection?
KBY-AI supports live detection, ensuring that the presence of a real, live person is verified during facial recognition processes.
Is KBY-AI’s Approach To Anti-spoofing User-friendly?
KBY-AI’s anti-spoofing solutions are crafted to be user-friendly, offering seamless integration and straightforward user interaction.
How Does KBY-AI Tackle 3d Mask Attacks?
KBY-AI uses depth analysis and texture detection to effectively differentiate between genuine faces and 3D masks.
What Technologies Power KBY-AI’s Solutions?
KBY-AI’s solutions are powered by a combination of artificial intelligence, machine learning, and computer vision technologies.
Can KBY-AI Operate In Real-time Applications?
Yes, KBY-AI is optimized for real-time applications, providing immediate authentication decisions with high accuracy.
How Does KBY-AI Stay Ahead Of New Spoofing Techniques?
KBY-AI stays ahead by regularly updating its algorithms with data from new spoofing techniques and incorporating ongoing research developments.
Conclusion
Embracing KBY-AI’s advanced face anti-spoofing techniques is a smart move for digital security. Their innovative methods offer robust protection against cyber threats. Investing in such technologies not only enhances safety but also builds trust in biometric systems. Stay ahead of fraudsters – prioritize your digital defense now.