Face Spoofing Detection Technology identifies fraudulent attempts to bypass biometric security systems. It differentiates between genuine human features and artificial replicas.
In today’s digital era, securing personal and organizational data against unauthorized access is crucial. Face Spoofing Detection is an advanced line of defense in biometric authentication, safeguarding against impersonation attacks. This technology utilizes sophisticated algorithms and machine learning to analyze the characteristics of a user’s face, ensuring that the entity trying to gain access is not a photo, video, mask, or a different substitute for an actual face.
With its importance escalating in various sectors, including banking, smartphone security, and access control systems, this technology is vital in preventing identity theft and maintaining high-security standards. As cybercriminals grow more clever, integrating robust Face Spoofing Detection has become imperative for a trustworthy validation process.
Introduction To Face Spoofing Detection Technology
The Rising Threat Of Digital Impersonation
Our digital world faces a growing menace: face spoofing. Bad actors use photos, videos, and masks to trick systems. They steal identities and access what should be secure. Everyone with a digital footprint could be a target. Recognizing this threat is step one in the battle for cybersecurity.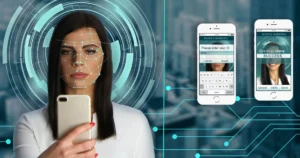
Role Of Detection Technology
Face Spoofing Detection technology acts as the guardian of our digital identities. It distinguishes real human features from fakes. Complex algorithms and machine learning come into play. They analyze facial patterns, texture, and depth. This technology doesn’t just detect; it evolves. Learning from new spoofing tactics, it adapts to keep our digital selves safe.- Live detection checks for natural human responses.
- Texture analysis finds differences between real skin and masks.
- 3D sensing discerns flat imagery from actual, dimensional faces.
Types Of Face Spoofing Attacks
Photo And Video Attacks
Photo attacks are simple yet effective. Here, the attacker uses a photograph of a valid user to deceive a system. Even high-resolution displays can present images that seem real enough to fool facial recognition. Video attacks step up the game. An attacker might use a video clip of the legitimate user. This can trick systems into ‘seeing’ a live person, due to the moving images.3d Masks And Models
With more advanced technology, criminals use 3D masks and models to spoof facial recognition. They create physical masks or head models that replicate the person’s face. These models can be so detailed that they fool systems designed to detect liveness or depth.Deepfakes And Synthetic Media
Deepfakes represent a sophisticated threat. Using artificial intelligence, attackers generate highly realistic images or videos. These can be almost undetectable to the human eye or older recognition systems. Synthetic media also include altered voices or facial expressions, which can create believable fake identities.Biometrics And Its Vulnerabilities
Today, we rely on biometrics to keep our data safe. Faces, fingerprints, and voices unlock phones and doors. Yet, smart tricksters find ways to break in. Let’s peek at how our digital locks might not be as strong as we think.
Importance Of Biometric Security
We use biometrics because they are unique to us. Unlike a password, no one else has your fingerprint or face. This makes it hard for thieves to sneak in. Think of biometrics as special keys that only you have. They keep your stuff extra safe.
Identifying Weaknesses In Facial Recognition
Even the best locks have weak spots. Facial recognition uses tech to look at your face and say “Yes, that’s you!”. But, if someone tricks the system with a fake face or mask, they might get past the lock. We need to find these weak points so we can fix them. Face Spoofing Detection Technology is our new guard. It spots fakes and calls them out. Like a smart detective, it looks for clues that don’t match your real face. This tech is learning every day to keep your stuff safer than ever.
- High-tech cameras: They scan for depth and warmth to tell if it’s the real you.
- Software smarts: Programs that check the face move in a real, not fake way.
- Quick updates: As tricksters get smarter, so does the software.
Technological Developments In Detection
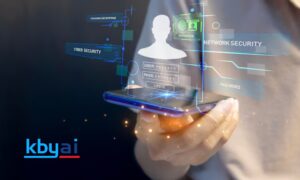
Evolution Of Detection Software
Early detection software relied on basic analysis. It checked for simple cues like movement or light. Modern solutions use advanced algorithms. They scan for depth, texture, and temperature. Latest programs use 3D modeling and AI to spot fakes. They compare new images with known real ones to find mismatches.- 2D Analysis: Early methods checked for photo attacks.
- 3D Detection: Now, software looks for lack of depth on masks.
- AI and Machine Learning: Newest systems learn from past attacks. They get better over time.
Integration With Existing Systems
Face spoofing detection technology is not standalone. It integrates with current security systems. This blend keeps systems updated without big overhauls.System | Integration Method |
---|---|
Mobile Devices | Embedded in OS for unlocking devices |
Banking Apps | Added as a layer for secure transactions |
Security Cameras | Paired with real-time monitoring software |
Methods Of Face Spoofing Detection
Motion Analysis Techniques
Motion analysis detects face spoofing by analyzing movements. Live faces exhibit natural motion like blinking and mouth movements.- Sequence analysis: Tracks face over time.
- Blink detection: Looks for natural eye blinks.
- Head movement: Notes how the head moves.
Texture Analysis
High-resolution images reveal texture patterns unique to live skin. Spoofing attempts often fail to replicate these details accurately.Texture Feature | Real Face Indicator |
---|---|
Fine skin details | Presence of pores and wrinkles |
Color patterns | Natural color variations |
Image quality | High-resolution textures |
3D Face Shape Analysis
A real face has depth and contours that a flat image cannot copy. 3D shape analysis spots differences in depth.- Depth sensors: Map the face’s 3D structure.
- Structured light: Projects patterns to measure contours.
- Stereo cameras: Use two viewpoints to gauge depth.
Machine Learning And Artificial Intelligence
Leveraging AI For Enhanced Security
AI drives innovation across many fields, and security is no exception. Harnessing the power of AI, systems can swiftly identify attempts at face spoofing. This technology works relentlessly, learning from each interaction. It improves its detection capabilities over time, staying steps ahead of fraudulent activities.- Real-time Analysis: AI examines live feeds to catch spoofing as it happens.
- Pattern Recognition: AI recognizes facial patterns, differentiating real from fake.
- Constant Improvement: AI adapts to new threats, enhancing its defense tactics.
Training ML Models To Recognize Spoofs
Training ML models is a complex task that requires patience and precision. First, researchers feed thousands of real and spoofed images into the system. The ML model learns to distinguish subtle differences that may not be visible to the human eye.- Data Collection: Gathering a diverse range of images to train the models.
- Feature Selection: Identifying which characteristics are most important for detection.
- Model Training: Teaching the AI how to recognize and respond to various threats.
Challenges In Detection Accuracy
Dealing With Real-world Variations
Real-world variations present significant challenges for face spoofing detection systems. Variations can include different lighting conditions, facial expressions, and angles. Even the presence of accessories such as glasses or hats can affect detection accuracy. These systems must be robust enough to handle these variations seamlessly.- Lighting Conditions: Changes in ambient light can alter the appearance of the face, leading to potential false rejections or acceptances.
- Facial Expressions: A smile or frown may significantly change the facial features that the detection system uses for verification.
- Angles and Orientation: A face captured at different angles can look vastly dissimilar, posing a challenge to the detection algorithm.
Adaptive Attack Strategies
Fraudsters constantly evolve their strategies to bypass face detection systems. They develop new spoofing techniques that can mimic real-life attributes even closer. This adaptive nature means that face spoofing detection systems must continually update to counteract these evolving threats.- Detection systems should always stay updated with the latest spoofing attack vectors.
- They need the ability to learn from attempted breaches to bolster their defense mechanisms.
- Continuous research and development are essential to stay ahead of sophisticated spoofing techniques.
Hardware Solutions
Specialized Cameras
Specialized cameras are at the forefront of tackling face spoofing threats. These cameras capture more than what meets the eye. They detect unique details invisible to standard cameras.- Infrared Cameras: Identify heat patterns on a human face.
- 3D Cameras: Map facial geometry, sensing depth to prevent 2D image attacks.
- High-resolution Cameras: Spot minute discrepancies on facial features.
Biometric Sensor Innovations
Biometric sensor innovations elevate the authenticity checks to new levels. These sensors are sophisticated and sensitive to detect subtle signs of life.Biometric Sensor Type | Function |
---|---|
Fingerprint Sensors | Analyze unique patterns for verified access. |
Retina Scanners | Scan eye patterns to confirm identity. |
Skin Texture Analysis | Check skin texture for additional proof. |
Software-based Approaches
Deployment In Consumer Devices
Integration of software-based technologies directly into consumer devices is a significant step towards fighting identity theft. Smartphones, tablets, and laptops have these systems built in. They ensure secure access to devices.- Facial recognition unlock systems on phones use these technologies.
- Banking apps use them for identity verification during transactions.
- Smart home devices apply this tech for personalized experiences.
Advancements In Algorithmic Methods
New algorithms improve how machines understand faces. These innovations increase detection accuracy and reduce chances of spoofing.- Machine Learning: Models learn from vast datasets to recognize spoofs.
- Deep Learning: Techniques dive deeper into image analysis, enhancing security.
- 3D Facial Mapping: Adds depth information to make spoofing harder.
- Liveness Detection: Confirms presence of a real, live person.
Liveness Detection – Face Spoofing detection
Concepts Of Liveness
Liveness detection, face spoofing detection separates real users from fakes. It finds unique signs of a living person. These signs can’t be copied by a photo or a video. They tell systems that the user is not just a picture. They are alive!- Movement: Live people can blink, smile or turn their head.
- Texture: Skin has certain patterns and reflections.
- Depth: Real faces have depth unlike flat photos.
- Response: Live people can follow prompts, like looking up.
Techniques For Ensuring ‘alive’ Verification
Various techniques make sure a user is alive. These techniques ask for actions or check for natural signs.Technique | Description |
---|---|
Active | Users perform actions, like blinking. |
Passive | Checks happen without user actions. |
Challenge-Response | Users respond to prompts on the screen. |
Texture Analysis | Software looks for skin features. |
3D Sensing | Cameras measure the face’s depth. |
Cross-dataset And Cross-domain Testing
Significance In Real-world Application
Face spoofing detection is a shield in technologies we use daily. ATMs, smartphones, and workplace entries rely on secure facial recognition. Systems trained on a broad range of data can identify diverse spoofing tactics. This means safer transactions and increased trust in biometric systems.- Banking security enhancements
- Smartphone authentication reliability
- Access control in sensitive areas
Improving Generalization Of Detection Models
A robust detection model excels in unseen environments. Cross-dataset and cross-domain testing are key. They expose models to a variety of spoofing attacks. By leveraging international datasets and operating across different backgrounds and lighting conditions, models learn to adapt. This results in a dynamic system that remains one step ahead of spoofers. Below are ways this testing strengthens face spoofing detection:Approach | Benefit |
---|---|
Diverse training data | Adapts to variable spoof attempts |
Multi-domain scenarios | Enhances detection in different settings |
Continuous learning | Evolves with emerging threats |
Industry Applications
Industry Applications: Safeguarding with face spoofing detection technology offers protection in many fields. It spots fake attempts to use someone’s face. Banks, border security, and hospitals use it a lot. It keeps services safe and secure. Let’s explore how different industries put this smart tech to work
Banking and financial sectors
Banking companies keep money safe with this technology.
- ATM Security: ATMs use cameras that tell real faces from fake ones.
- Mobile Banking: Users can log in with their face, and the tech checks it’s really them.
- Fraud Prevention: The tech finds frauds trying to steal by using a photo or mask.
Border Control And Law Enforcement
Border guards use it to make sure people are who they say they are. It checks faces at airports and borders quickly and accurately. Officers find fake documents fast. This helps catch criminals who change their looks.
Health Care and access management
Hospitals make sure only the right people get into secure areas.
- Staff log in with their face for quick access.
- Patient identification becomes super accurate.
- It stops anyone from pretending to be a doctor or nurse.
Future Trends In Face Spoofing Detection Technology
Predicting The Next Generation Of Spoofing
Future systems will not just react, they’ll predict. They’ll use large datasets and machine learning. This boosts their ability to spot fakes.- Enhanced AI Algorithms
- Real-time Behavioral Analysis
- Rapid Response Protocols
Preemptive Defense Mechanisms
The future is about stopping attacks before they happen. Soon, defense systems will:- Monitor security 24/7
- Analyze threats in real-time
- Deploy countermeasures proactively
Aspect | Current Tech | Future Trends |
---|---|---|
Learning | Reactive Analysis | Predictive Modelling |
Response | After Detection | Real-time Blocking |
Defense | Passive Protection | Active Counteraction |
User Education And Awareness
Importance Of Informed Users
Informed users become the first line of defense against face spoofing. They know what to do and what to avoid. Users aware of the risks are less likely to fall for traps set by attackers. Educated users also help protect entire systems by following best security practices.Strategies For Public Education
Public education on spoofing detection aims to empower users. It makes technology safer for everyone. Strategies include:- Workshops: Organizations can host interactive sessions.
- Social Media Campaigns: Quick tips and facts reach a broad audience.
- Online Tutorials: Detailed guides help users understand spoofing.
- Simulation Exercises: Real-world scenarios test user knowledge.
Regulatory And Ethical Considerations
Balancing Privacy With Security
Prioritizing safety is essential, but it shouldn’t infringe on an individual’s right to privacy. Developers and implementers of face spoofing detection systems must focus on creating solutions that protect both public safety and personal privacy.- Minimize data collection to what’s necessary for verification
- Implement robust encryption to protect user data
- Ensure transparent user consent protocols are in place
- Develop clear data storage and deletion policies
Compliance With Global Standards
As companies operate globally, compliance with international regulations is non-negotiable. Global standards guide the ethical use of biometric technology.Standard | Purpose | Region |
---|---|---|
GDPR | Data Protection | Europe |
CCPA | Consumer Privacy | California, US |
ISO/IEC 19795 | Biometric Performance Testing | International |
User Experience And Usability
Maintaining Convenience
The best security solutions must be convenient. Users want quick and easy access to their devices and services. Face Spoofing Detection Technology can offer that. Let’s see how:- No extra steps: Users can unlock devices with just a glance.
- Fast processing: Advanced algorithms quickly analyze facial features.
- Seamless integration: This tech works with existing apps and systems without hassle.
Building Trust With Users
Trust is a cornerstone of any technology adoption. Users need to feel confident in the Security measures protecting them. Face Spoofing Detection Technology does this well:Feature | Benefit |
---|---|
Real-Time Alerts | Notifies users of any unauthorized attempt. |
Data Privacy | Personal information stays safe and secure. |
Consistent Updates | Technology evolves to remain ahead of threats. |
Impact Of Covid-19 On Detection Technology
Shifts In Biometric Security
Biometric security, like fingerprint scanners, had to change. With germs a concern, touching surfaces posed risks. Face spoofing detection offered a hygienic substitute. Companies adopted it to maintain security without physical contact.Acceleration Of Contactless Verification
COVID-19 sped up the use of contactless verification. This tech uses cameras to scan faces quickly and safely. It’s used in phones, offices, and banks. No need for touching any device. It means fast, clean checks for entry and transactions.Frequently Asked Questions For Face Spoofing Detection Technology
What Are The Techniques Used In Face Spoofing Detection?
Face spoofing detection techniques include photo attacks, video replay attacks, 3D mask creation, and deepfakes leveraging advanced graphics and artificial intelligence.
What Technology Is Used For Facial Recognition?
Facial recognition technology typically utilizes AI and machine learning, particularly deep learning algorithms like convolutional neural networks (CNNs). Advanced software processes biometric data to identify facial features.
How Does Face Anti-spoofing Work?
Face spoofing detection technology detects fake facial features by analyzing the uniqueness of a user’s physical face. It uses algorithms to differentiate between a real person and a non-live face, such as masks or photos, by examining the depth and texture of facial features.
Can Facial Recognition Systems Be Spoofed?
Yes, facial recognition systems can be fooled by techniques such as using photos, masks, or videos, challenging their security measures.
Conclusion
As we navigate the evolving landscape of digital security, face spoofing detection stands as a vital defense. By embracing the latest advancements, individuals and organizations can effectively shield themselves from cyber threats. Implementing robust detection systems is not just smart; it’s crucial for ensuring the integrity and trust in our technological interactions.
Let’s prioritize security and stay one step ahead of malicious actors.